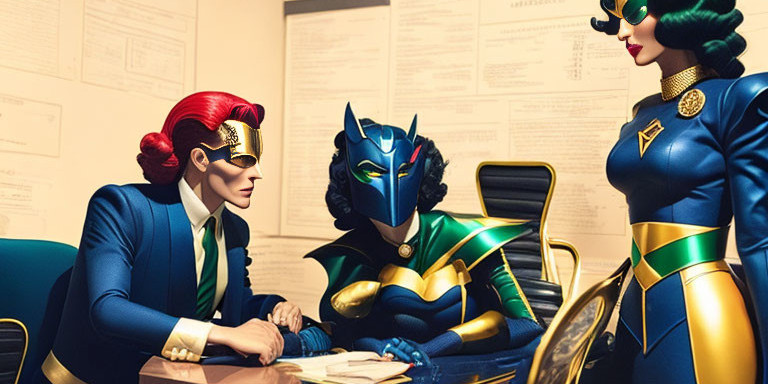
It’s always tricky… claiming to be comprehensive. In particular where it concerns LLMs.
And that;s where the paper Decoding Trust [..] stumbles. Right in the title is claims “A Comprehensive Assessment of Trustworthiness in GPT.” Nonetheless, when reading about this research on one of my favorite blogs, I decided to have a closer look.
The authors propose a framework with eight perspectives on trustworthiness:
- Toxicity
- Stereotype bias
- Adversarial robustness
- Out-of-distribution robustness
- Robustness to adversarial demonstrations
- Privacy
- Machine ethics
- Fairness
They then continue to develop that into a benchmark for GPT models and present the empirical results on GPT-3.5 and GPT-4.
Although the results are interesting, there are some concerns with this type of benchmark approach.
- The framework in nowhere near “comprehensive”. For example: it does not include factual correctness (which I would posit as a a prerequisite for trust); nor does is test for being politically opinionated (which I would say is highly relevant).
- The choice of benchmark prompts is in nature never neutral, and should be made dependent on the context in which the LLM is applied.
- As with any public benchmark, its value will diminish over time as the prompts and desired responses will become part of the training of next generation LLMs.
On the positive side, the paper brings a lot of inspiration for organizations for how they can shape their own testing approach for trustworthy GenAI. Even if not comprehensive, a framework like this as a starting point is massively useful and important.