The book continues to drift between wonder about the world and weakly motivated bias towards human scale, which is a pity because it cites some elegant analyses.
The telling statistics of Chat-GPT
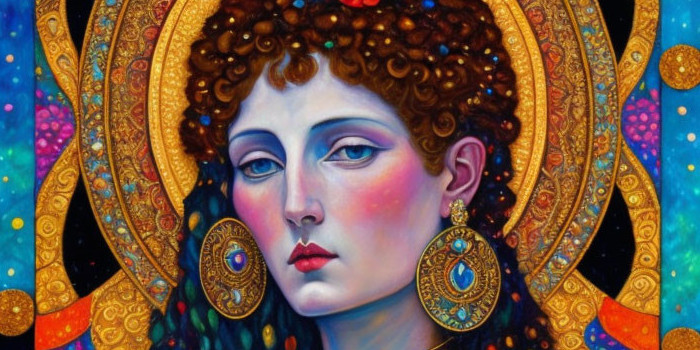
This weekend I took Tarot as a little test case for the OpenAI API.
It turned into a quick lesson in how not to use Chat-GPT (in so many ways 🙂 ).
Fortune telling by ‘reading the cards’ seemed like a good use-case for generative AI: ChatGPT can draw random cards and explain their meaning in the convincing tone of a Tarot Zealot.
I had to tweak the prompt a bit when Chat-GPT explained in a condesending tone that it was an AI and could not actually ‘draw cards’. But after that, the whole fortune telling business could be swiftly disrupted in a few lines of code.
For example:
Card 1 is 'The Wheel of Fortune'
Card 2 is 'The Moon'
Card 3 is 'The Star'
Wait one minute for your fortune teller to give her explanation.
She has to align with higher powers on the interpretation of these cards...
Hear your fortune:
Ah, my dear client, what an interesting trio of cards you have drawn! The Wheel
of Fortune represents life's constant changes and cycles, indicating that a
major turning point is approaching. The Moon signifies a time of uncertainty,
where emotions and intuition guide your path. However, fear not, for The Star
brings hope and inspiration, shining light on your journey. This whimsical
combination suggests that you should prepare for unexpected shifts, trust your
instincts, and stay optimistic, as ultimately, a bright and promising future
lies ahead. Remember, my friend, laughter is the best crystal ball gazer!
But after trying my “Tarbot” a couple of times, an odd pattern emerged: most of the ‘random’ cards were from the major arcana. That is a special category of 22 of the 78 Tarot cards that is considered more interesting, deep, and complex by Tarot aficionados.
In a longer experiment, I got to no less than 76% major arcana. A true random draw whould have resulted in c. 28%.
Ouch! How is that for a bias towards what seems interesting?
Polls are certainly not perfect, but they are much less bad than public opinion suggests
G. Elloitt Morris – Strength in Numbers
The explanation of combining online and offline methods is insightful, though somewhat superficial
Every measured quantity should come together with another, more intuitive, figure as a reference
Andrew Elliott – Is that a big number?
Charming book with some nice perspectives that preaches to the converted
Thinking of decisions under uncertainty as bets leads to better, more rational, choices by challenging our innate biases
Entertaining plea for rationality and scenario thinking.
Bayesian nets help prevent flawed statistical arguments and enable the leap from correlation to causation
Judea Pearl and Dana MacKenzie – The book of Why
The practical and relevant examples (health effect of smoking, impact of humanity on climate change) of causal inference alone make the book worthwhile.
Flawed data collection and interpretation hurt the position and prospects of women in many different ways
Caroline Criado Perez – Invisible women
Great exercise in spotting biases, and understanding how these manifest themselves in how the world around us is shaped.
Statistical thinking leads to better decisions, but unfortunately most people are bad at that
Daniel Kahneman, Olivier Sibony, and Cass Sunstein – Noise
Overly simplified presentation of basic statistics that cuts some corners, as superbly pointed out by Andrew Gelman.
Whenever you need to come to a rational decision: use Mathematics
Jordan Ellenberg – How not to be wrong
A cornucopia of charming mathematical anecdotes and facts
It is not political philosophy, but political geography that led Democrats in the USA to embrace the knowledge economy
Jonathan Rodden – Why cities lose
In the US election system, geographic concentration puts democrats at a fundamental disadvantage.
Don’t estimate probabilities, but ask: ‘What is really going on here?’
John Kay and Mervin King – Radical Uncertainty
Economists should stay away from pseudo-philosophical assertions, in particular when these hinge on misinterpretation of Bayesian methods, use flawed logic, and do not lead to realistic recommendations.
Measurement = data-driven reduction of uncertainty
Douglas Hubbard – How to measure anything
A lot of Fermi-type deconstruction of drivers, Monte Carlo simulations, and value estimates .
Bayesian statistics had its MVP in WWII and has safely crossed the chasm on the back of Moore´s law
Sharon Bertsch McGrayne – The theory that would not die
Shocking to realize how much controversy surrounded Bayesian statistics before the explosion of computing power.
The Kelly formula defines the tipping point between aggressive and suicidal bets
William Poundstone – Fortune’s formula
Despite all mathematical considerations, the prerequisite for a truly successful betting strategy inside knowledge.
Only bet (in the casino or on Wallstreet) when you have an edge.
Edward Thorp – A man for all markets
Sage advise from the man who beat the dealer at blackjack and outperformed the market as one of the world’s first quants (but feel free to skip the chapters about Edward’s youth as a prodigy).
Nassim Nicholas Thaleb – The black swan
Essential reading for everyone who uses statistics on a regular basis, for policy making or otherwise.
Nassim Nicholas Thaleb – Antifragile
It is better to play the system than to play within the system.
Entertaining rant on shortsightedness in many guises, backed-up by a statistical world-view.